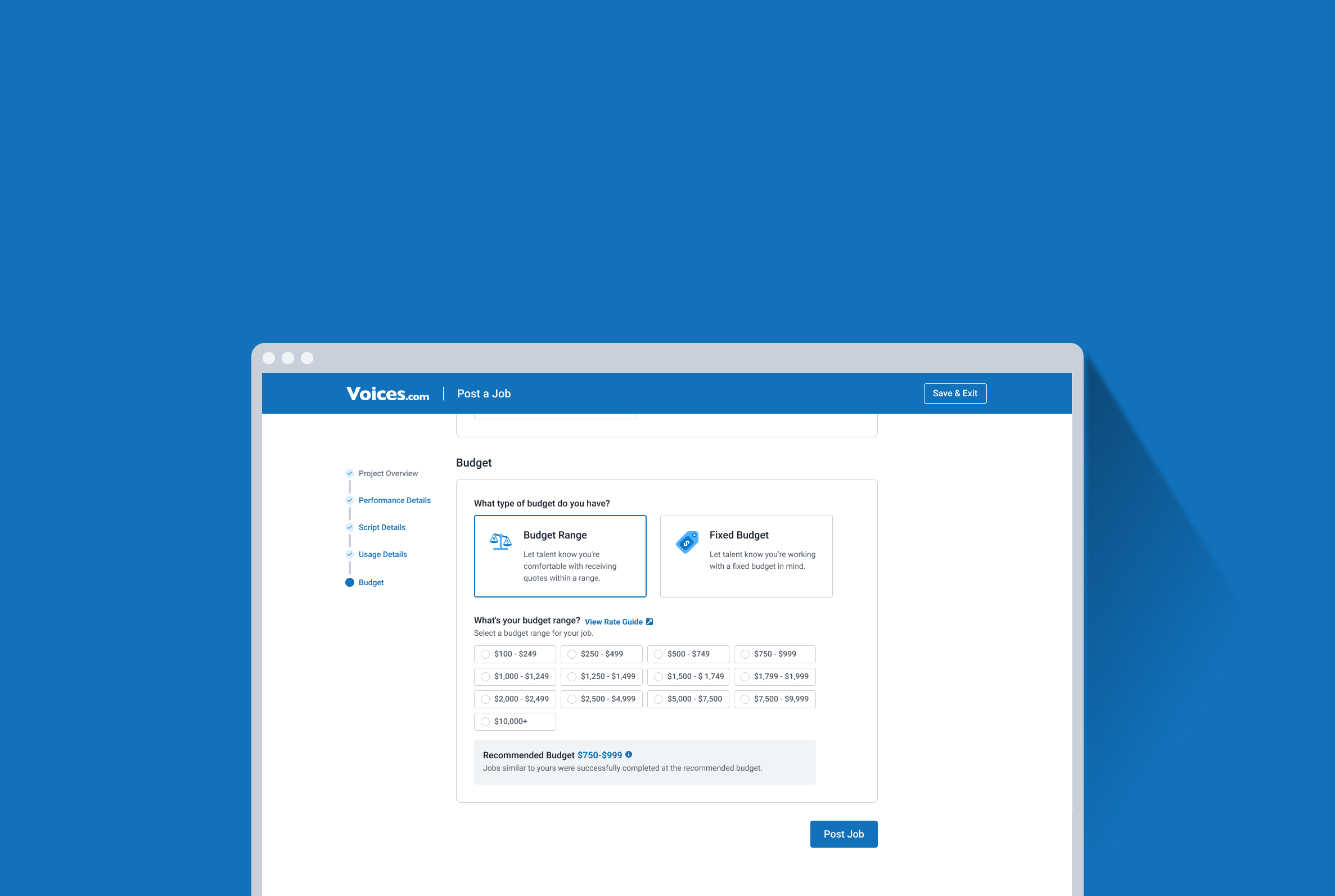
Fair Job Pricing
Fair Job Pricing
Through research we had found that Clients often struggle with confidently setting a price for their Voice Over projects. Whether it’s early in the creative planning process, or once the user reaches the job form on our platform, there seems to be an underlying level of uncertainty as it relates to pricing VO. We wanted to help reduce the experienced uncertainty for clients while maintaining fair job prices for our talent users.
Preview of the design sprint board. Made in Whimsical.
Proposed Solution
During the discovery phase of this project, we decided that we wanted to implement a machine learning engine on our job posting form. The machine learning algorithm would use historical data and job details to recommend a budget that is most likely to lead to the job being hired. Details like the usage rights, language, length, and word count would affect the predicted recommended budget.
Our goal for the front-end UI was to inform, encourage and educate clients with our pricing recommendation. We wanted to make sure that clients had enough information to make informed pricing decisions, regardless if they choose to use our recommendation.
As the lead designer, I prepared and facilitated a design sprint for the working team. During this design sprint, we aligned the team on the challenge, discussed best practices, reviewed competitors for inspiration, and sketched solutions. We left the design sprint with three design variations we wanted to prototype and test with our client users.
Research
We conducted usability testing with 3 design variations with 9 participants. The participants' experience on our platform ranged from: Net-new users, Experienced Platform Users, and Inexperienced Platform Users. During our usability sessions we were looking for design variation preference, how likely users would be to listen to the recommended budget, when and why they would not use the recommendation and what would make them more likely to use the recommendation.
Through testing we discovered a preference for inline styling over validation or our smart pricing options. Clients also shared with us a strong desire for more data and numerical feedback, as it increased their trust in the recommendation and us as a platform.
Three design variations we showed during usability testing.
“I feel like I know (how to price), but I have doubts when it comes to different usages that I’m unfamiliar with”
— Usability Participant’s Quote
Final Solution
The final design solution was to position the recommendation as a banner within the budget section. This treatment allowed us to provide timely help to our client users when selecting a budget for their jobs. We wanted to highlight and encourage use of the recommendation, rather than force clients to use the recommendation. In this banner we display the recommended price, either as a range or as a single value based on the budget type selected. If we did not have enough information to provide a recommendation, we provided a direct link to the platform’s rates and pricing guide for additional help.
Results & Learnings
We released the first iterations of the pricing recommendation engine as an A/B test for 4 months. During this time, the new design (B variant) saw a 3.3% increase on GSV and a 0.5% increase to our hire rate. After the A/B test was complete, we switched all users to the new job posting form, with the recommended pricing engine.
-
A second iteration of the pricing recommendation has since been released through an A/B test. For the second iteration, we wanted to focus on making the recommendation more usable, integrated and interactive while improving client’s confidence when pricing their jobs. Following similar steps as the first iteration, the team participated in a design sprint (lead by myself), conducted usability testing with client users and is monitoring results through a A/B test on our platform.
Second iteration currently in A/B testing. This iteration focused on making the recommendation a selectable option. Our hypothesis is that by making it easy to select the recommended option, we can save our clients time and cognitive load - while increasing the feature’s adoption rate.